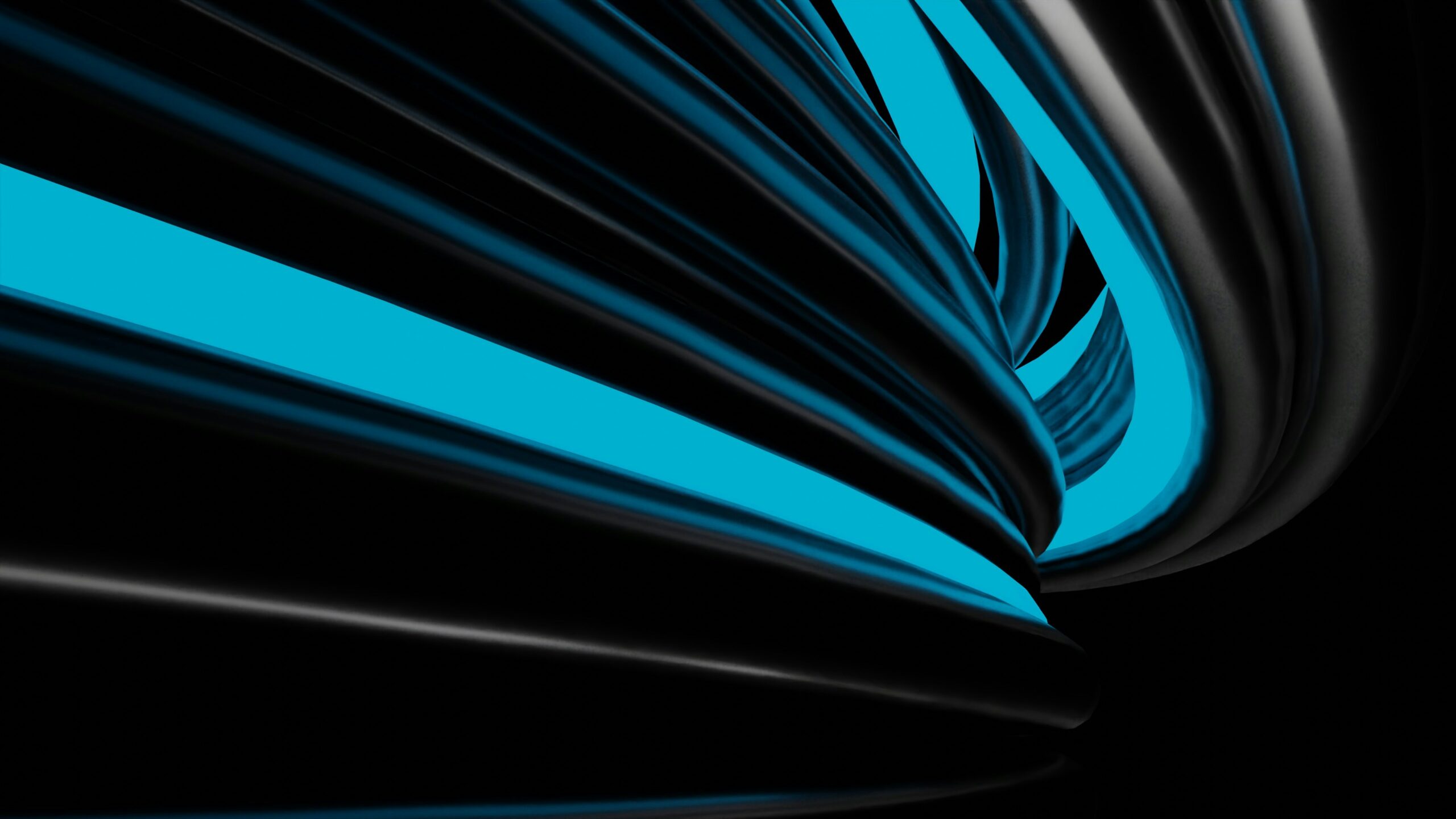
Small Language Models (SLMs) are quickly becoming the go-to choice for businesses seeking AI that’s cost-effective, secure, and easy to deploy. While large language models (LLMs) like GPT-4 make headlines, most organizations don’t need billions of parameters or hyperscale infrastructure. They need practical AI.
SLMs deliver just that: performing targeted tasks with lightweight infrastructure and total data privacy.
So, what does SLM mean, and how can your business use them?
What Does SLM Mean?
SLM stands for Small Language Model, a lightweight AI system designed for specific tasks with fewer parameters than its larger LLM counterparts. SLMs typically range from a few hundred million to a few billion parameters.
That’s significantly smaller than today’s top-tier models’ 70+ billion parameter giants. But don’t let the size fool you. These models are fast, highly specialized, and capable of running on personal devices, edge servers, or right-sized private cloud environments.
In fact, open-source SLMs like Phi-2, Mistral, and LLaMA 3 8B can be fine-tuned for your exact use case. No hyperscaler GPU cluster required.
And the momentum is only accelerating. According to Gartner’s April 2025 forecast, organizations will use small, task-specific models three times more than general-purpose LLMs by 2027.
SLMs vs LLMs: What’s the Difference?
While both use similar transformer-based architectures, their purpose, cost, and performance profiles are quite different. Choosing the right model depends on your business needs, not just model size.
Here’s a quick comparison:
Feature | Large Language Models (LLMs) | Small Language Models (SLMs) |
Model Size |
10B+ parameters | <10B parameters |
Hardware Requirements |
High-end GPUs or TPUs | Run on local devices or modest cloud VMs |
Latency |
Often high due to cloud inference | Low (can run on edge or private servers) |
Data Privacy |
Data typically leaves the local network | Fully local or private cloud processing |
Use Case Fit |
General-purpose, multi-domain | Narrow, domain-specific tasks |
Cost to Run |
High (GPU, bandwidth, inference fees) | Low (CPU-friendly, open-source models) |
Deployment Flexibility |
Requires cloud/hyperscaler dependencies | Deployable on-prem, cloud, or hybrid |
For a deeper dive into the architectural and cost differences, check out this detailed breakdown on SLMs vs. LLMs.
What Do SLMs Mean for Your Business?
Whether you’re building an internal chatbot, adding AI to a SaaS product, or streamlining document processing, SLMs offer key advantages:
- Lower cost of deployment on CPUs or entry-level GPUs
- No vendor lock-in when run on your own infrastructure
- Faster response times when deployed locally
- Greater data privacy with on-device or single-tenant hosting
These traits make SLMs ideal for industries like healthcare, finance, retail, education, and manufacturing—where security, compliance, and speed are critical.
What Are the Real-World Applications of SLMs?
The shift from general-purpose LLMs to task-specific SLMs is already transforming the way we use AI. This shift is helping businesses understand SLM’s meaning beyond the acronym, seeing how these models can bring real-time AI to classrooms, edge devices, and secure industries.
Education: Personalized Learning with Khanmigo
Khan Academy’s Khanmigo tutor is a standout example. Built with small language models, it gives students personalized feedback, encourages critical thinking, and adapts to individual learning styles, all while keeping data usage private and controlled by schools.
Software Development: Local AI Coding Assistants
Engineers and hobbyists are using lightweight open-source models like Phi-2 or LLaMA 3 Mini to run local AI agents for coding support, logic checking, and error debugging. Tools like LM Studio or platforms like Ollama enable on-device AI assistance with no cloud dependency.
Wearables and Edge Devices
Small models are even being integrated into smart glasses, phones, and vehicles. For example, Meta’s Ray-Ban smart glasses are beginning to use compact models for real-time translations and AI interactions, all processed at the edge.
Healthcare and Compliance-Heavy Industries
Doctors, financial analysts, and legal professionals are testing models that run directly on secure tablets or air-gapped servers. These setups enable SLMs to process sensitive data while keeping it fully contained within the organization’s environment.
To explore these use cases in depth, check out this excellent video by Ragnar Pichla, where he demos real-world SLM applications from offline AI assistants to on-device coding copilots.
Why HorizonIQ is the Right Home for Your Lightweight AI
At HorizonIQ, we can help you go from “What does SLM mean?” to full deployment—guiding you through model selection, infrastructure, and security best practices. Our private cloud offerings are purpose-built for:
- Single-tenant data isolation
- GPU or CPU-backed compute options
- Full control over model selection and environment configuration
- Flexible deployment across Proxmox or VMware infrastructure
You don’t need a hyperscaler to build lightweight AI. You need infrastructure that fits your vision and your budget.
SLM Meaning: The Future of AI is Smarter, Not Bigger
SLMs are not scaled-down versions of LLMs, they’re optimized for the real world. They give you the power of AI, without the sprawl of oversized infrastructure or the risk of data leakage.
From smart tutoring to offline language translation, the future of AI is already here. And it fits in your pocket.
Looking to build a secure, scalable AI stack around small language models? Explore how we support real-world AI with cost-effective infrastructure.