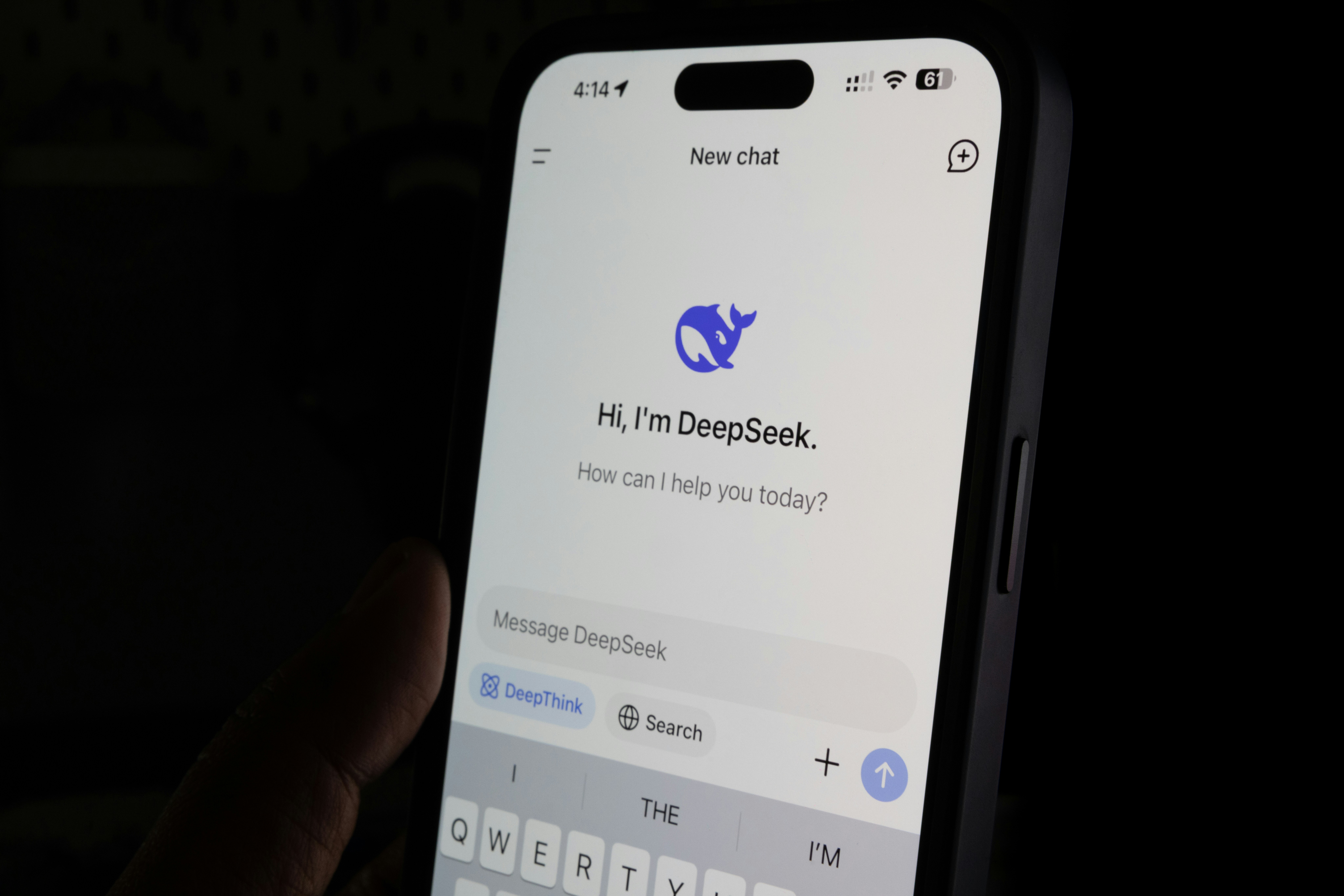
The AI industry is advancing at breakneck speed, and DeepSeek has recently made waves—and crashed stock markets—by claiming it trained a powerful AI model for just $5.6 million.
This is a fraction of what it costs to develop similar AI models in the U.S., which can range from $100 million to $1 billion. This raises an important question: Will DeepSeek change the way AI models are trained and, in turn, transform the data center industry?
What is DeepSeek?
DeepSeek is a Chinese AI company specializing in open-source large language models (LLMs). Its flagship model, DeepSeek-V3, powers an AI assistant that has gained significant traction, surpassing OpenAI’s ChatGPT in popularity.
A key differentiator of DeepSeek’s technology is its cost efficiency. While major AI firms like Meta train models on supercomputers with over 16,000 GPUs, DeepSeek-V3 was supposedly trained using only 2,000 Nvidia H800 GPUs. At what was claimed to be over 55 days at a fraction of the cost.
Despite this leaner infrastructure, its performance is competitive with leading AI models, excelling in answering complex queries, logic problems, and programming tasks. DeepSeek’s rapid rise has sparked global interest—with some viewing it as a challenge to U.S. AI dominance.
It has also faced scrutiny, with OpenAI investigating whether DeepSeek trained its model using proprietary ChatGPT data. Additionally, several countries, including Australia, Italy, Taiwan, and the U.S., have restricted DeepSeek’s use in government sectors due to security concerns.
Despite these challenges, DeepSeek’s ability to develop high-performing AI at a lower cost has positioned it as a disruptive force.
Is DeepSeek’s Cost Factor A Game Changer?
Training AI models requires massive computational resources, which has led many companies to need thousands of GPUs running in high-performance data centers.
The industry norm has been to pour billions into AI infrastructure, with companies like OpenAI, Google, and Meta investing heavily in specialized data centers to support their AI ambitions.
DeepSeek’s claim that it trained a cutting-edge model at a fraction of the cost challenges this assumption.
If true, it suggests that AI models might not need the same scale of hardware investment as previously thought. This could impact:
- The demand for high-performance GPUs
- The need for expansive data center facilities
- The electricity consumption required to train AI
Companies spending billions on AI infrastructure might reassess their investments if AI training becomes significantly cheaper and more efficient.
The fact that AWS, Azure, and others have already released Deepseek into their cloud platforms demonstrates a significant shift in the industry towards more cost-effective and scalable solutions.
What Is DeepSeek’s Impact on Data Center Growth?
AI-driven data center expansion has been one of the biggest trends in tech. Nvidia, the leader in AI chips, has seen skyrocketing demand as companies race to build AI infrastructure.
The belief has been that more AI equals more data centers, more power consumption, and more hardware.
DeepSeek’s approach could challenge this logic in two ways:
- Less Need for Massive Compute: If AI models can be trained with fewer GPUs and at a lower cost, companies may not need to scale up their data centers at the same pace. This could slow down new data center construction projects.
- Shift Toward Smaller AI-Focused Data Centers: If AI models become more efficient, we may see a rise in smaller, more localized AI processing hubs instead of massive, centralized data centers.
However, there’s another possibility: If DeepSeek’s model makes AI training more accessible, more companies might enter the AI space, increasing the overall demand for data centers.
Is DeepSeek’s AI and Energy Consumption a Turning Point?
Training large AI models consumes enormous amounts of electricity, which is why power companies and data center operators have been preparing for a surge in energy demand.
If DeepSeek’s model truly operates with significantly lower power requirements, this could ease some of the concerns surrounding AI’s impact on global energy grids.
This could have multiple effects:
- Lower costs for AI startups: If AI models don’t require extreme power consumption, it could reduce entry barriers for smaller companies.
- Less reliance on hyperscale data centers: More efficient models could lead to a preference for distributed, modular data centers instead of massive hyperscale facilities.
- Shift in energy infrastructure investments: Power companies anticipating higher AI-related energy demand might need to reconsider their projections.
But there’s a catch: If AI adoption accelerates due to lower costs, overall energy consumption could still rise, even if individual models become more efficient.
What Is The Skepticism Around DeepSeek?
While DeepSeek’s claims are impressive, some industry experts, including Elon Musk, are skeptical. One major concern is whether DeepSeek had access to more GPUs than it is letting on. If DeepSeek secretly used significantly more compute resources, then its cost claims may not be entirely accurate.
Another concern is transparency. AI models trained in China often face scrutiny due to privacy, data security concerns, and censorship. Ask DeepSeek chat anything that may be viewed as controversial about China, and you’re bound to get this response:
Also, the reported $5.6 million cost does not necessarily include all prior research, data acquisition, or other hidden expenses. If these costs were excluded, the real expense of training DeepSeek’s model could be much higher.
What Does This Means for the Future of AI and Data Centers?
DeepSeek’s breakthrough raises more questions than answers. If its model truly represents a new, cost-effective way to train AI, it could disrupt the industry by:
- Reducing the need for hyperscale AI data centers
- Making AI more accessible to smaller companies
- Lowering energy consumption for AI processing
- Shifting investment strategies in AI infrastructure
However, if its claims turn out to be overstated, the AI industry may continue on its current path—one of increasing GPU demand, larger data centers, and skyrocketing energy consumption.
One thing is certain: The AI landscape is evolving rapidly. Whether DeepSeek is a disruptor or just another hype, its emergence signals that the industry is moving toward more efficiency and cost-conscious AI development.
The future of AI and data centers will depend on how these advancements play out in real-world applications.